DCT-Net: Domain-Calibrated Translation for Portrait Stylization
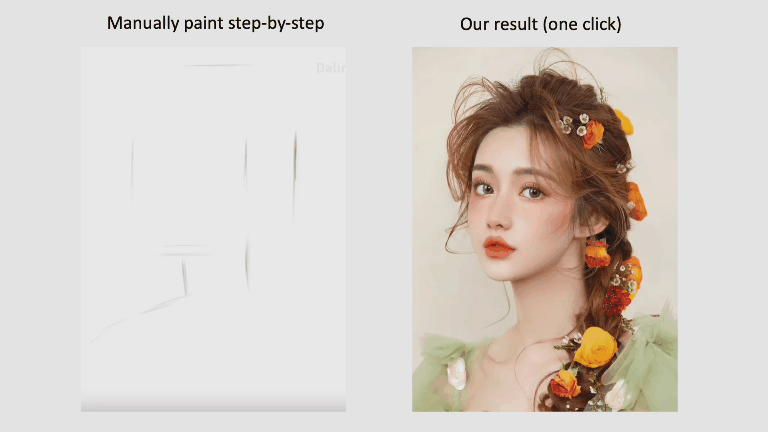
Abstract
This paper introduces DCT-Net, a novel image translation architecture for few-shot portrait stylization. Given limited style exemplars (∼100), the new architecture can produce high-quality style transfer results with advanced ability to synthesize high-fidelity contents and strong generality to handle complicated scenes (e.g., occlusions and accessories). Moreover, it enables full-body image translation via one elegant evaluation network trained by partial observations (i.e., stylized heads). Few-shot learning based style transfer is challenging since the learned model can easily become overfitted in the target domain, due to the biased distribution formed by only a few training examples. This paper aims to handle the challenge by adopting the key idea of "calibration first, translation later" and exploring the augmented global structure with locally-focused translation. Specifically, the proposed DCT-Net consists of three modules: a content adapter borrowing the powerful prior from source photos to calibrate the content distribution of target samples; a geometry expansion module using affine transformations to release spatially semantic constraints; and a texture translation module leveraging samples produced by the calibrated distribution to learn a fine-grained conversion. Experimental results demonstrate the proposed method's superiority over the state of the art in head stylization and its effectiveness on full image translation with adaptive deformations.
Core idea
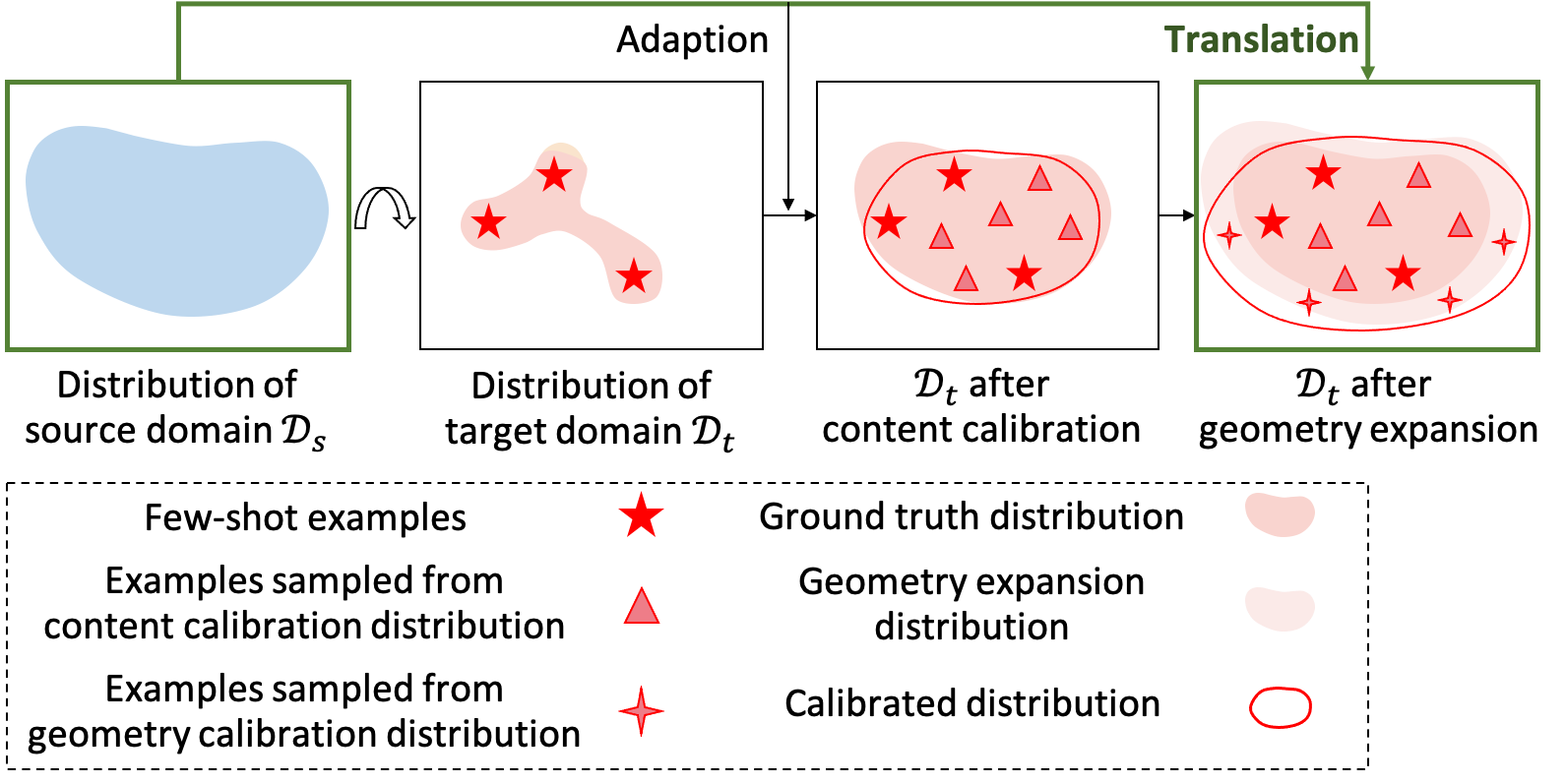
Fig.2 An illustration of domain-calibrated translation.
Networks
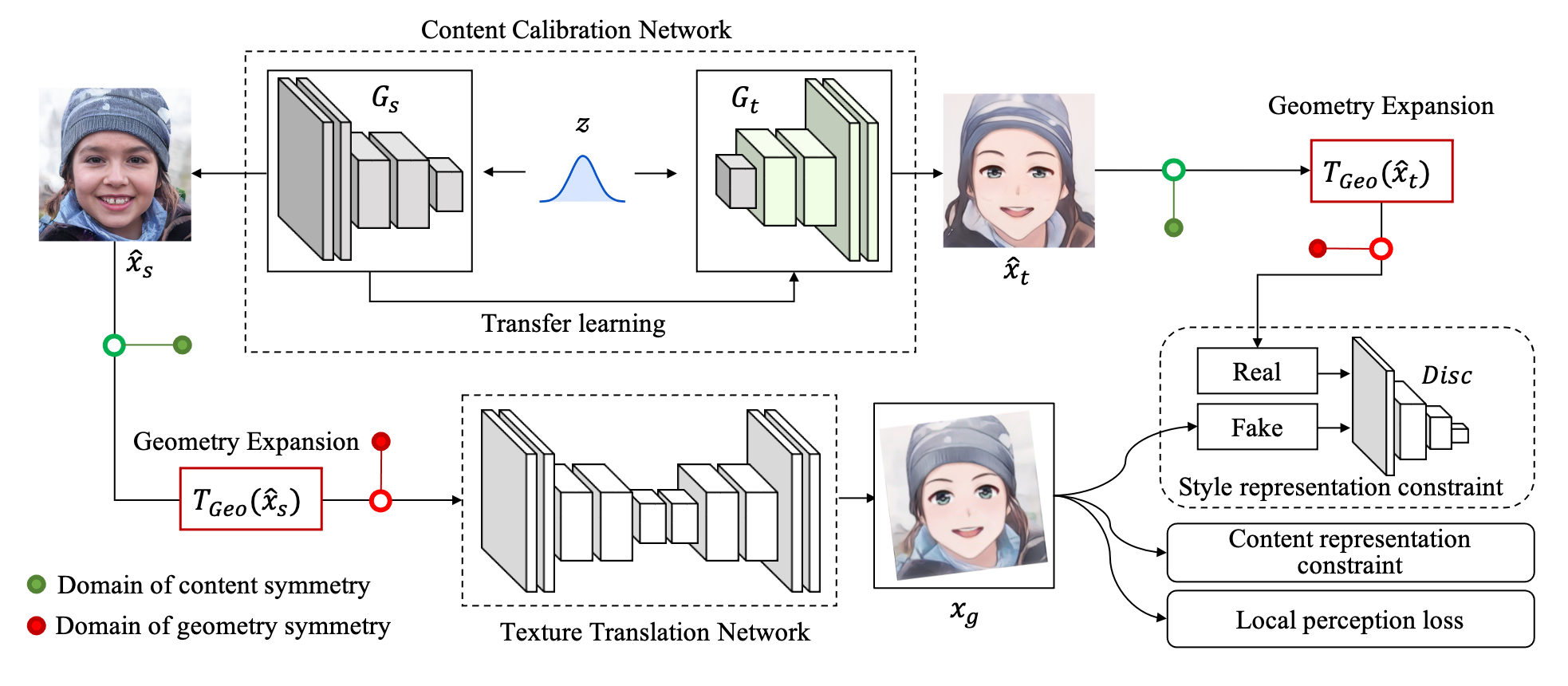
Fig.3 An overview of the proposed framework.
Demo Video
Citation
@inproceedings{men2022dct, title={DCT-Net: Domain-Calibrated Translation for Portrait Stylization}, author={Men, Yifang and Yao, Yuan and Cui, Miaomiao and Lian, Zhouhui and Xie, Xuansong}, journal={ACM Transactions on Graphics (TOG)}, volume={41}, number={4}, pages={1--9}, year={2022}, publisher={ACM New York, NY, USA} }